Disadvantages of AI in Cybersecurity: Risks You Need to Know
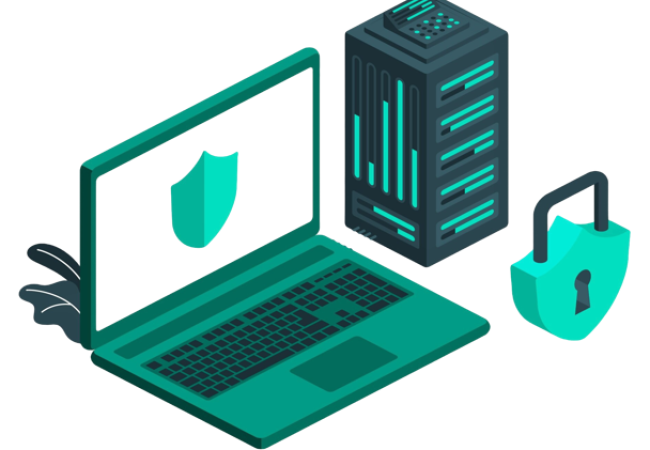
Artificial Intelligence (AI) has become a transformative force in cybersecurity, offering tools that can analyze threats, predict attacks, and automate responses faster than ever before. From real-time threat detection to proactive vulnerability management, AI promises to revolutionize how businesses defend themselves in an increasingly digital world.
However, alongside these advantages come significant challenges and risks. While AI enhances cybersecurity capabilities, its implementation and reliance introduce vulnerabilities that businesses cannot afford to overlook. In this article, we’ll explore the disadvantages of AI in cybersecurity, highlighting why it’s crucial to balance innovation with caution when integrating AI into your security strategy.
High Implementation and Maintenance Costs
Implementing AI in cybersecurity is not only about adopting cutting-edge technology but also about making significant investments. AI systems require substantial financial resources for initial setup, including hardware, software, and skilled personnel to develop, train, and maintain them. The costs don’t stop at implementation; regular updates, retraining of models, and infrastructure scaling to accommodate evolving threats can quickly add up.
Moreover, AI solutions often demand specialized expertise, which can be expensive and hard to find. Companies need data scientists, AI engineers, and cybersecurity professionals who understand how to integrate and optimize AI systems effectively. For small to medium-sized businesses (SMBs), these high costs can make AI adoption impractical, leaving them dependent on traditional, less effective cybersecurity measures.
Without a clear return on investment, the financial burden of AI in cybersecurity might outweigh its benefits, especially for organizations with limited budgets or resources. This makes cost a significant disadvantage that organizations must consider before committing to AI-driven cybersecurity solutions.
Lack of Transparency (Black Box Issue)
One of the major disadvantages of AI in cybersecurity is its lack of transparency, often referred to as the “black box” issue. Many AI systems, particularly those based on deep learning, operate in ways that are not easily understood, even by experts. These systems make decisions and detect threats through complex algorithms and vast datasets, but the rationale behind their outputs is often unclear.
This lack of transparency poses significant risks. For instance, if an AI system flags a legitimate activity as a threat (a false positive), security teams may struggle to understand why it happened or how to prevent similar issues in the future. Conversely, if the AI fails to detect a threat (a false negative), it can leave systems exposed without clear insight into the oversight.
The black box issue can also undermine trust. Security teams and decision-makers may hesitate to rely on AI-driven tools if they cannot explain or justify the system’s decisions, especially in industries with strict compliance requirements. Regulatory bodies often demand detailed reporting and accountability, which can be challenging when using opaque AI systems.
To mitigate this challenge, businesses must prioritize explainable AI (XAI) solutions. However, these are still in development and may lack the maturity and accuracy of traditional AI systems, further complicating the adoption of transparent cybersecurity tools.
Dependence on Data Quality
AI in cybersecurity relies heavily on high-quality data for accurate threat detection. Poor, incomplete, or biased datasets can result in missed vulnerabilities, false positives, and flawed decisions. Additionally, attackers may exploit this dependence through “data poisoning,” introducing corrupted data to mislead AI systems.
Maintaining data quality requires continuous updates and retraining, which can be resource-intensive. Without proper data management, AI systems risk becoming outdated, reducing their effectiveness against emerging threats.
Risk of Adversarial Attacks
Adversarial attacks exploit vulnerabilities in algorithms by introducing deceptive inputs that can mislead detection systems. Hackers use these techniques to bypass security measures or create false alarms, effectively manipulating the AI’s decision-making process.
For example, crafted adversarial samples might evade malware detection or disrupt access control systems. Combating these risks requires ongoing model optimization, advanced safeguards, and additional resources, making it a persistent challenge for organizations relying on AI in cybersecurity.
Lack of Human Judgment
While machine learning models and automated systems can analyze vast amounts of data, they lack the ability to apply human intuition and judgment in complex situations. In cybersecurity, this is a critical issue.
For example, AI can identify unusual patterns or behaviors within a network, but it cannot always comprehend the nuances of the context. What may appear as a threat could, in fact, be a legitimate action or a false alarm. This absence of human insight can lead to over-reliance on automation and potentially overlook subtle, yet important, indicators of a cyber attack.
Thus, while AI can assist in detecting threats, human expertise remains crucial in interpreting results and making final decisions to ensure a balanced, effective security strategy.
Over-reliance on Automation
Relying too heavily on AI and automation in cybersecurity can lead to potential risks, especially when human intervention is necessary. While AI systems excel in processing vast amounts of data and detecting known threats, they may not be equipped to handle complex or novel cyber attacks effectively. Over-reliance on automated tools can result in missed threats or improper responses. Cybersecurity often requires adaptability and judgment, which automated systems may lack, increasing the risk of overlooking sophisticated attack methods or creating vulnerabilities. Balancing automation with human oversight is essential for an effective cybersecurity strategy.
Privacy Concerns
AI’s role in cybersecurity often involves extensive data collection and analysis, raising significant privacy concerns. As AI systems process large volumes of personal and sensitive data, there is a risk of data breaches or unauthorized access. Additionally, AI-driven security measures, such as facial recognition or behavioral tracking, can lead to ethical dilemmas related to user privacy. Striking a balance between robust cybersecurity and protecting individual privacy is crucial. Companies must ensure their AI tools comply with privacy regulations and adopt transparent data-handling practices to mitigate these concerns.
Conclusions
AI has the potential to transform cybersecurity, but it comes with notable risks that cannot be ignored. High implementation costs, lack of transparency, dependence on data quality, and vulnerability to adversarial attacks all present significant challenges. Additionally, the absence of human judgment, over-reliance on automation, and privacy concerns must be addressed to ensure a balanced and secure approach. By understanding these disadvantages, businesses can make informed decisions about integrating AI into their cybersecurity strategies and take necessary precautions to mitigate these risks.