QVQ-72B-Preview: Enhancing Visual Reasoning in AI
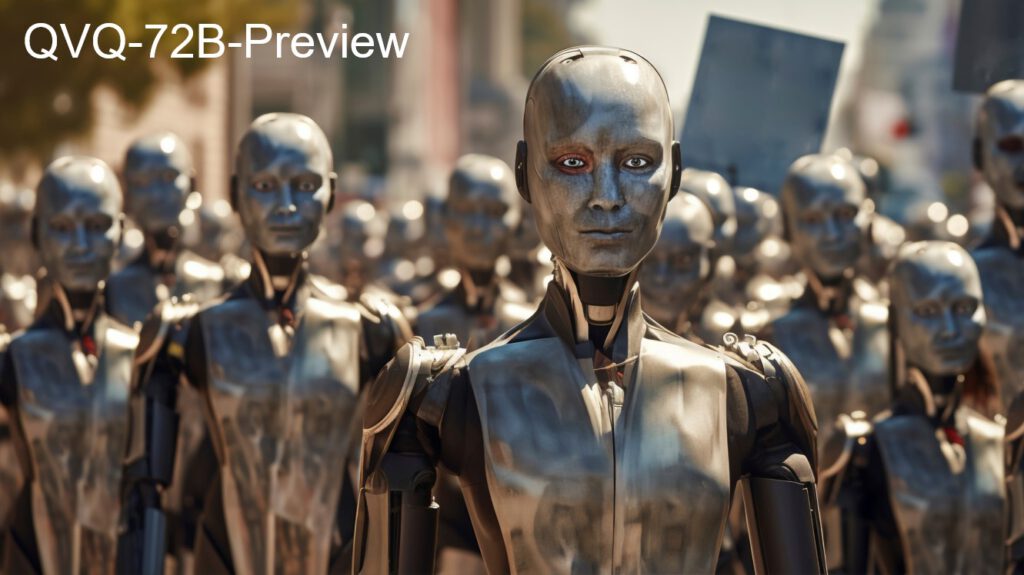
What is QVQ-72B-Preview?
QVQ-72B-Preview is an advanced multimodal AI model developed by the Qwen team. It enhances visual reasoning by integrating language and visual data. The model builds on the architecture of Qwen2-VL-72B but introduces significant improvements to tackle more complex reasoning tasks that require both text and image comprehension.
It leverages a transformer-based design, enabling it to process large amounts of data efficiently and accurately. QVQ-72B-Preview can understand and analyze visual content alongside natural language. This makes it a powerful tool for tasks requiring both verbal and visual reasoning, such as image interpretation, visual question answering, and multimodal problem-solving.
QVQ-72B-Preview is freely available on Hugging Face, allowing anyone to access and utilize it for research or applications.
Performance Benchmarks: QVQ-72B-Preview, DeepSeek V3, and GPT-4o
When comparing the performance of QVQ-72B-Preview, DeepSeek V3, and GPT-4o, each model excels in different areas. QVQ-72B-Preview stands out due to its strong multimodal capabilities, combining both visual and text-based reasoning, making it highly suitable for tasks that involve image understanding alongside text.
QVQ-72B-Preview outperforms GPT-4o and DeepSeek V3 in benchmarks requiring multimodal reasoning and visual problem-solving, such as MMMU (70.3) and MathVista (71.4).
DeepSeek V3 excels in pure text-based tasks like MMLU (88.5) and Code-related benchmarks but lacks advanced multimodal abilities.
GPT-4o performs well in natural language understanding and general reasoning but is limited when it comes to integrating visual data.
Data was taken from report and deepseek.com.
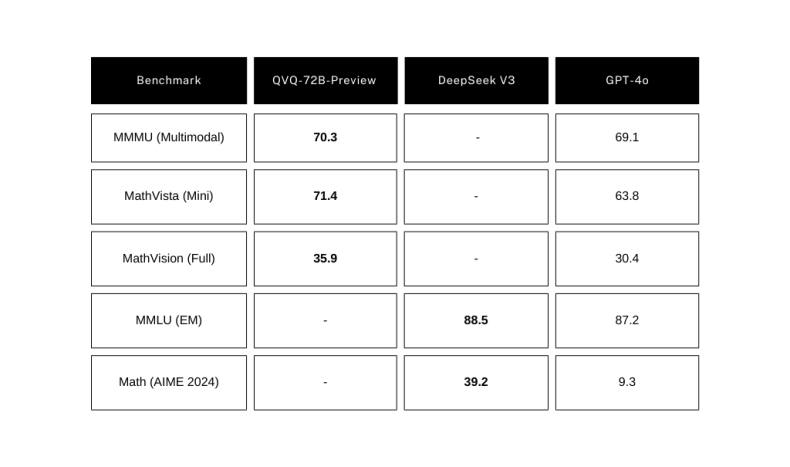
In summary, QVQ-72B-Preview is particularly useful for multimodal tasks, where visual and text data need to be processed together, while DeepSeek V3 and GPT-4o shine in their respective strengths—text-based tasks and general language understanding.
Applications of QVQ-72B-Preview
QVQ-72B-Preview is ideal for tasks that require the integration of both visual and textual information, making it suitable for various applications:
- Multimodal Reasoning: It can analyze complex problems that involve both images and text, such as interpreting scientific diagrams and solving physics problems that involve visual data, such as diagrams.
- Mathematical Problem Solving: The model effectively solves math problems that include visual elements, such as graphs and geometric diagrams, making it a powerful tool for education and research.
- Scientific Research: It helps interpret data visualizations and supports researchers in easily analyzing complex scientific diagrams and graphs.
- Educational Tools: QVQ-72B-Preview enhances learning in subjects such as physics, engineering, and mathematics by combining visual and textual explanations, making these topics more accessible.
Limitations and Challenges of QVQ-72B-Preview
While QVQ-72B-Preview offers groundbreaking advances in multimodal AI, it also encounters some limitations and challenges that need to be addressed for wider adoption and effectiveness:
Language Mixing and Code-Switching: The model occasionally struggles to maintain consistent language use, leading to unintentional language mixing or code-switching. This can affect the clarity of its responses in multilingual contexts.
Recursive Reasoning: QVQ-72B-Preview sometimes exhibits circular reasoning, resulting in overly detailed answers that may not provide definitive conclusions. This can affect the overall efficiency of problem-solving.
Safety and Ethical Concerns: As with many advanced AI systems, ensuring safety and reliability is of paramount importance. Additional safety measures are required to ensure safe and ethical use in various applications.
Performance Limitations: Although QVQ-72B-Preview shows strong performance in visual reasoning, it still has issues compared to its predecessor, Qwen2-VL-72B-Instruct. The model occasionally loses focus on the image content, leading to inaccurate or irrelevant results, a phenomenon known as “hallucinations.”
Conclusions
QVQ-72B-Preview marks a significant step forward in the field of multimodal AI as it excels at tasks that require both visual and textual reasoning. Its impressive performance in benchmarks such as MMMU and MathVista underlines its potential to revolutionize areas such as scientific research, education and complex problem solving.
In the future, the model promises further improvements in multimodal integration and real-world applications. Addressing current challenges, such as recursive thinking and improving concentration in multi-step visual tasks, will be central to the further development of the model. With these refinements, QVQ-72B-Preview could become an indispensable tool in a variety of industries, providing more reliable and accurate solutions to complex, multimodal problems.